Men are overrepresented in senior academic positions in Economics. What factors can explain this phenomenon, and how can we make the academic environment more inclusive?
Women in economics
Why is this article in English?
This article is part of our English publication ‘Women in Economics’. This dossier is in English because English is the main language of the economics and business faculties in the Netherlands, so an ESB dossier about the people who work there should be in English.
In brief
– Gender bias and meritocratic beliefs explain men’s overrepresentation in senior academic positions.
– Performance evaluation practices that appear to be meritocratic often result in unequal distributions of success.
– Several practical, evidence-based, interventions can mitigate bias and promote inclusion in academia.
Men are overrepresented in senior academic positions in Economics (Teunissen and Hogendoorn, 2018). While gender inequality in academia is universal (Miller et al., 2015), it is especially pronounced in the Economics discipline (Leslie et al., 2015) and in the Netherlands in particular (Miller et al., 2015). In nearly four decades, only six women have ever made it into the ESB Economics Top 40.
It is important to note that promoting gender equality is not just a matter of fairness; it is – as should be of interest to Economists – also a matter of efficiency. For instance, Hsieh et al. (2018) have argued that no less than a quarter of the economic growth in the US between 1960 and 2010 can be attributed to what they call “the improved allocation of talent” of members of underrepresented groups. For the Netherlands specifically, The McKinsey Global Institute recently calculated that greater gender parity in labor force participation, STEM fields, and senior positions, would add more than 100 billion euros to Dutch GDP (McKinsey, 2018).
To shed light on this phenomenon and to present insight into possible interventions, we provide a conceptual and empirical analysis of the factors underlying gender differences in career advancement in Economics, drawing on the latest research in the behavioural sciences.
Causes of underrepresentation
Empirical evidence on the causes of women’s underrepresentation in senior positions points to gender stereotypes more than women’s preferences and ability. Gender stereotypes are commonly accepted ideas about the roles of men and women in society, both at home and at work. These ‘received ideas’ do not only reflect what men and women typically do, but also what they should do and should not do, and in that sense are both descriptive and prescriptive (Heilman, 2012; Ridgeway, 2009). The predominant expectation is that men work and women care; these societal gender roles are translated into typical attributes of men (e.g. competitive and assertive) and women (e.g. kind and modest).
Stereotypes may, or may not, reflect reality in terms of actual differences between men and women. What is important though is that “stereotypes, whether ‘accurate’ or not, function as expectations, thus guiding perceptions and judgment” (Biernat and Sesko, 2018). For math-intensive disciplines such as Economics, it is pertinent that many people (both men and women) believe that men have a higher innate ability for math than women (Leslie et al., 2015). In reality, there is much more overlap than difference in the distribution of the actual math abilities of men and women. However, even when members of groups collaborating on math tasks are informed that the woman in the group is the person with the highest math ability, team members still tend to put more confidence in the men in the team, as a result hampering actual group performance (Van Dijk et al., 2018).
Gender bias (or prejudice) is a cognitive distortion that follows from a lack of stereotype fit between stereotypes of a particular social role and gender roles. In senior roles, there is a greater degree of stereotype fit for men than for women (Heilman, 2012). Women are thus ‘presumed incompetent’ or even inferior to men when it comes to senior, often male-dominated, roles. It is important to realise that both men and women are biased – both favour men in senior roles (Koenig et al., 2011). In academia, the stereotypical successful academic is competitive and assertive, whereas women are expected to be modest (Bleijenbergh et al., 2013). In the case of Economics, the famous adagio “think manager, think male” (Koenig et al., 2011; Schein, 1973) is thus likely translated into “think economist, think male”.
Stereotypes about gender and science start young and are strong (Miller et al., 2018) – especially in Economics (Leslie et al., 2015) and in the Netherlands (Miller et al., 2015) – which makes gender bias a common phenomenon in this particular context. Making counter-stereotypical educational and professional ‘choices’, such as moving into a math-intensive field, is harder and generates more disapproval from observers and evaluators than fitting the stereotype does. Without changing stereotypes, encouraging young women to choose such disciplines will not have much effect on their representation at higher levels. In addition, women in senior positions who show assertive and self-promoting behaviour may experience backlash for not adhering to the injunctive norm of modesty fitting in with their stereotypical gender role (Rudman, 2008).
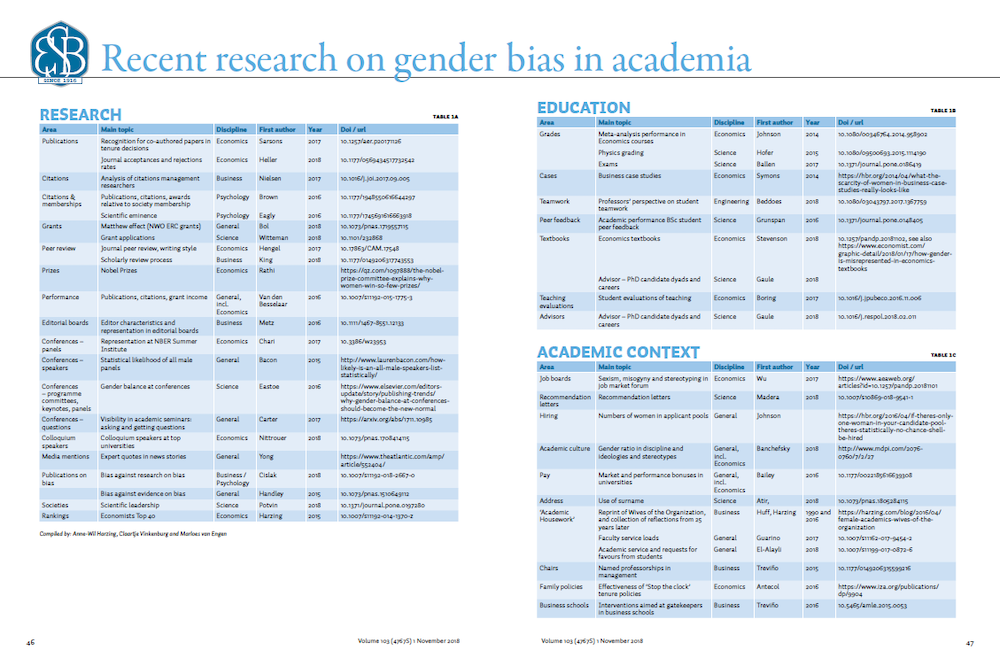
Click on the image to download the infographic
Consequences of gender bias
As gender bias is often implicit and subtle, it is more difficult to recognise and thus harder to counter than blatant and explicit discrimination (Biernat et al., 2011). Bias colours both decision making and the application of criteria for selection and promotion (Vinkenburg, 2017), resulting in fewer promotions for women and ultimately in the underrepresentation of women at senior levels, relative to men. Martell et al. (2012) refer to mathematical simulations to show that only a little bit of bias in every performance evaluation along the way results in considerable gender segregation in senior positions. In terms of individual careers in academia, stereotypes and bias in performance evaluation lead to a vicious and difficult to break cycle in which women receive fewer opportunities to develop into high-performing researchers (Van den Besselaar and Sandström, 2017).
A significant part of the existing evidence of gender bias in academic hiring and promotion decisions comes from experimental studies using either fictional CVs or real CVs in which only the name and matching gender is changed (Moss-Racusin et al., 2012; Milkman et al., 2015). Even with an identical track record, the CV of job applicants with male names is usually preferred, and men have a far higher chance of being selected and/or promoted than women.
Academic selection and promotion
What makes it hard to pinpoint gender bias in actual academic selection and promotion decisions is that this is only possible if we can control or correct for objective performance, which in academic careers is usually measured by publications, citations and grant income. Recent studies that were able to do so show that gender bias is clearly present. Following a cohort of Dutch researchers who submitted an application for an NWO Veni (Veni grant awarded by the Netherlands Organisation for Scientific Research) early career grant between 2003–2005, Van den Besselaar and Sandström (2016) found that men’s careers progressed faster than women’s. This was true across disciplines (including Economics), even when controlling for differences in year group, performance and mobility.
Sarsons (2017) finds that one reason why women in Economics get tenured at a lower rate than men, even with similar academic performance, is that women receive less credit than men for articles that are co-authored with men. However, even when actual performance data is available and candidates are equally qualified, decision makers have been shown to overestimate men’s track records relative to women’s (King, 2006). In grant applications, women are equally successful to men when reviewers evaluate the research idea, but not when they evaluate the researcher’s CV (Van der Lee and Ellemers, 2015; Witteman et al., 2018).
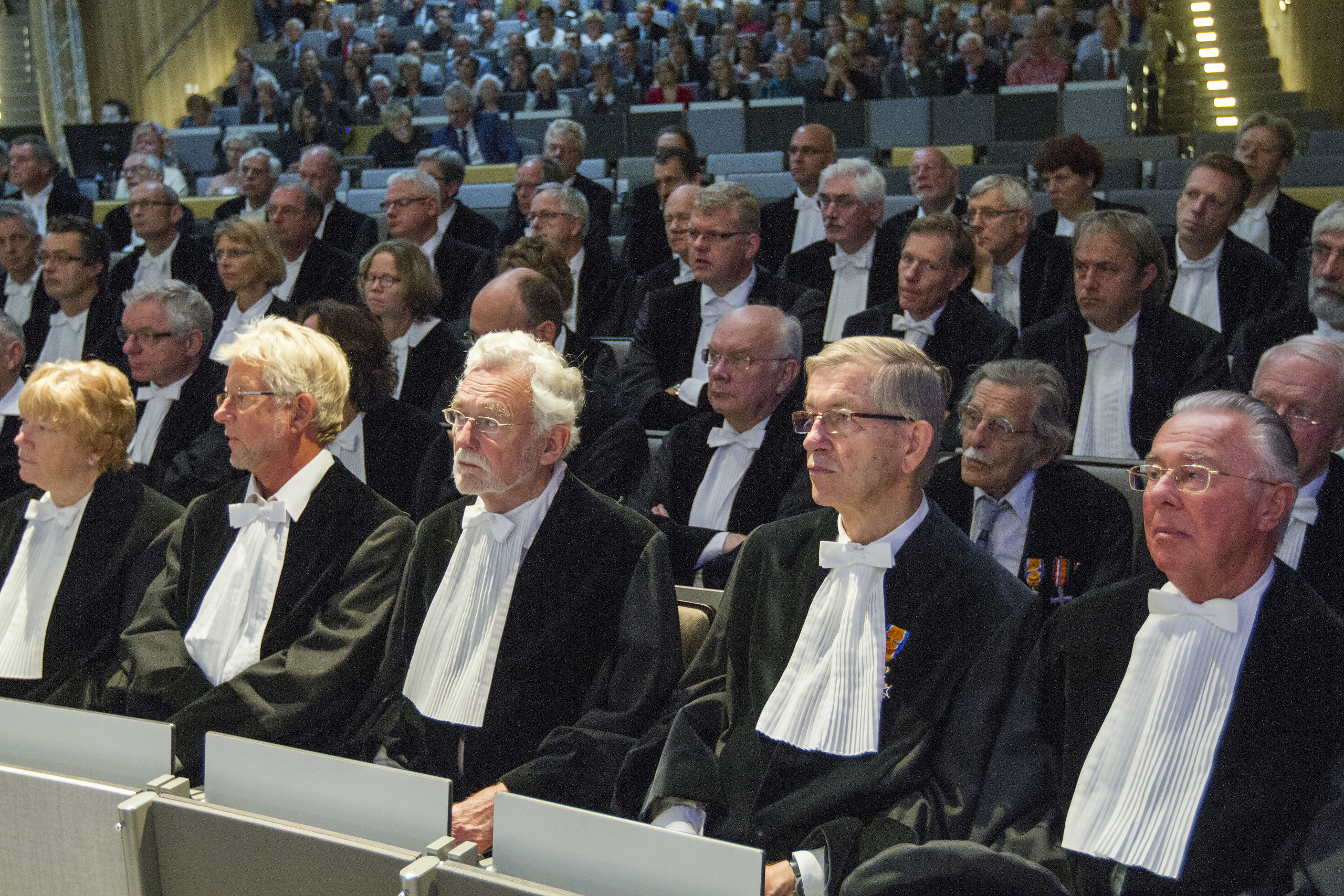
Meritocracy and decision-making
The extent to which academics accept evidence of gender bias is complicated by their strong belief in, and matching rhetoric of, meritocracy (Nielsen, 2016). We all like to believe that those who are successful in academia are so because they have more merit (i.e. worth, superior quality) than those who are not successful, and that everyone has an equal chance regardless of their gender, race, class, or other non-merit factors (Castilla and Benard, 2010). However, reward allocation and performance evaluation practices that appear to be meritocratic (Joshi et al., 2015) often result in an unequal distribution of success in favour of some, regardless of the actual distribution of merit (Vinkenburg, 2017). For instance, when both the actual number of and relative contribution to publications are overestimated for male academics, counting publications favours men relative to women. Academia suffers from the paradox of meritocracy; in systems with strong meritocratic beliefs, decision makers are ironically more biased in favour of men (Castilla and Benard, 2010). To stay with the same example: counting publications appears to be objective, and thus justifies the system and its unequal outcomes.
Where decision makers have discretion, bias is more likely to affect their decisions (Castilla, 2015). However, (partial) formalisation of selection and promotion processes to reduce ambiguity and discretionary space has mixed effects (Abraham, 2017). Quantifying performance by counting publications and citations may only serve as a threshold for candidates to be considered, with the ultimate selection or promotion decision being based on other, less objective and more bias prone criteria including potential and fit (Vinkenburg, 2017). Finally, efforts to make decision makers in academia aware of the existence of bias and its cumulative disadvantageous effects on women’s careers often lead to resistance, denial, and even anger (Handley et al., 2015; Moss-Racusin et al., 2015; Van den Brink, 2015). However, when efforts to de-bias the decision-making process are successful, more women are hired and promoted (Devine et al., 2017).
‘Bias collection’ and recommendations
In order to move the needle on women’s representation in senior positions in academia, we present two collections: First, collated evidence of gender bias, and second, practical and evidence-based interventions to mitigate bias and to make academia more inclusive. The ‘bias collection’ (see Table 1a–1c) brings together very recent empirical evidence related to gender differences on a range of indicators relevant to academic careers. Indicators range from publications, citations and grant applications, questions at conferences, student evaluations, and recommendation letters, to ‘academic housework’. The two boxes present a list of practical, evidence-based interventions. These interventions do not directly target stereotypical notions of what a successful academic career in Economics in the Netherlands looks like. However, the resulting, more balanced, representation of women and men at the top of the academic hierarchy will ultimately affect stereotypes and reduce bias. Provided the willingness to engage in change is there, the interventions are all relatively simple to implement, do not require significant financial resources and come with the added benefit of making our workplaces and professional environments more inclusive, without compromising high standards.
Box 1: Performance evaluation and decision-making interventions
* Ensure the use of objective and transparent metrics. ‘Citizen bibliometrics’, facilitated by Google Scholar, Microsoft Academic, and Publish or Perish (Harzing, 2007) have made it easier for every academic to compare themselves to others in terms of both publications and citations, and to do so using a variety of data sources.
* Use a variety of performance indicators. In rankings of academics, different types of indicators favour different groups. For instance, citation-based rankings show different results from publication-based rankings, such as the ESB Economics Top 40. Two alternative Economics Top 40s (Harzing and Mijnhardt, 2015), based on authorship-corrected citation metrics rather than on publications, featured three and five women respectively, including two in the top 4 and the top 6 respectively, whereas in nearly four decades only six women ever made it to the publication-based ESB Economics Top 40.
* Change principles of authorship ordering. Economics is one of the few disciplines that favour alphabetical ordering over contribution-based ordering. As shown by Sarsons (2017), ordering by level of contribution will benefit women. Alphabetical order should thus be reserved for publications where contribution was truly equal.
* Compensate for time to care in performance evaluation. Managers and evaluators need to be attentive to the structural conditions affecting women’s and men’s publication rates and compensate for time to care. Given the propensity of temporary contracts, shared care responsibilities and part-time work for both women and men in Dutch academia, this type of compensation takes into account the realities of combining career and care, and sustains academic career ambitions (Vinkenburg et al., 2015). For example: Tilburg School of Economics and Management offers research resources (e.g. reduction of teaching load, research assistance, travel grants) covering for time lost because of compulsory pregnancy leave; VU SBE (VU School of Business and Economics) adapts publication criteria for employees working part-time (factoring in FTE).
* Introduce more transparency and accountability in both selection decisions and the performance evaluation process as a means to reduce gender bias (Castello, 2015). Limiting discretion in these processes can be supported by for instance developing algorithms for automatic promotion (Bosquet et al., 2018) or by using lottery thresholds for grant applications (Fang and Casadevall, 2016).
* Introduce behavioural modification programmes for selection and promotion of committee members that monitor and provide feedback over a longer time (Devine et al., 2017), such as customised bias mitigation sessions (Vinkenburg, 2017). These sessions focus on optimising the decision-making process through the operationalisation and application of criteria for performance and potential.
Box 2: Workplace interventions
* Engage in Participatory Modelling, a system dynamics-based intervention in which senior decision makers (e.g. dean and department chairs) together identify issues in, and solutions for, the career advancement (or stock and flow) of women and men in their faculty. This method has been applied at Dutch and other European universities and has resulted in several evidence-based local interventions to promote gender equality (Bleijenbergh and Van Engen, 2015; Van Arensbergen et al., 2017).
* Offer more flexibility and longer paid leave (Goldin, 2014). In the Dutch context that would include longer mandatory paid paternity leave, to decouple the stereotypical notion that mothers care and fathers work, and to reduce ‘defaulting’ into part-time work.
* Create women-only academic networks. Although several universities in the Netherlands have institutional, cross-disciplinary networks for women, networks such as CYGNA (Harzing, 2014) that are cross-institutional, but within-discipline might provide a more fruitful platform for mutual support, learning and networking.
* Ensure substantive representation in all spheres of academia, i.e. decision-making boards including student associations, applicant pools, conference panels, internal and external communication, and even pictures on the (virtual and real) wall. A simple rule of thumb is 50/50, as having only one token woman simply makes her the exception to the rule and does not change stereotypes (King et al., 2010).
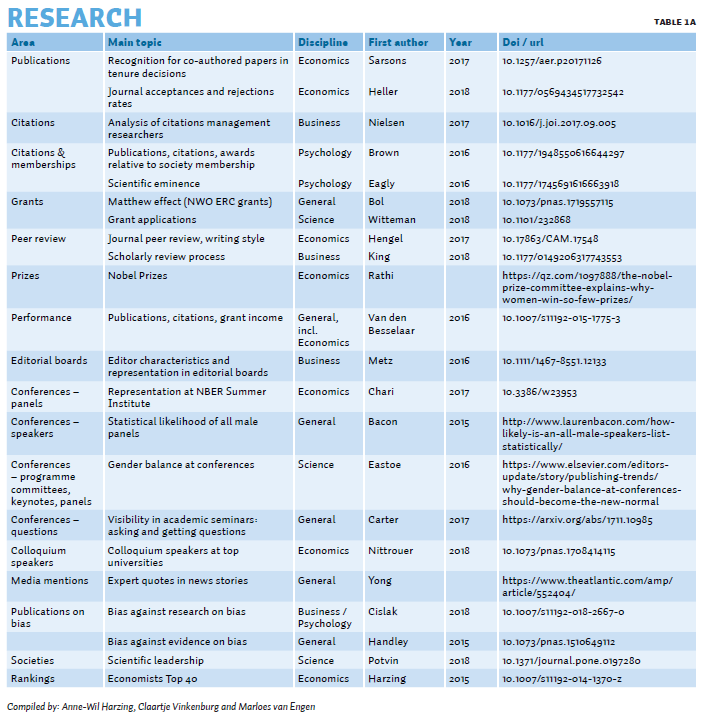
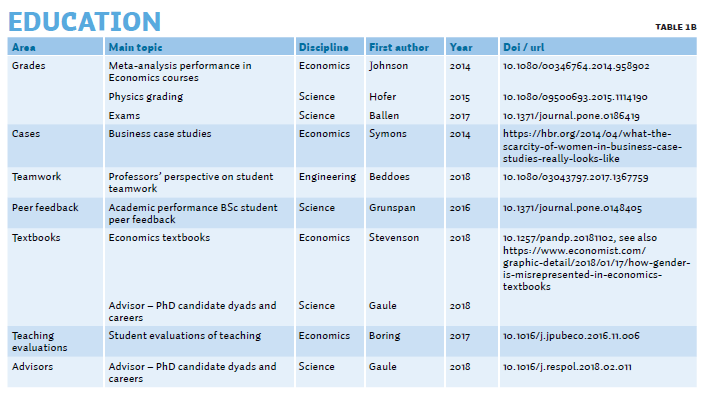
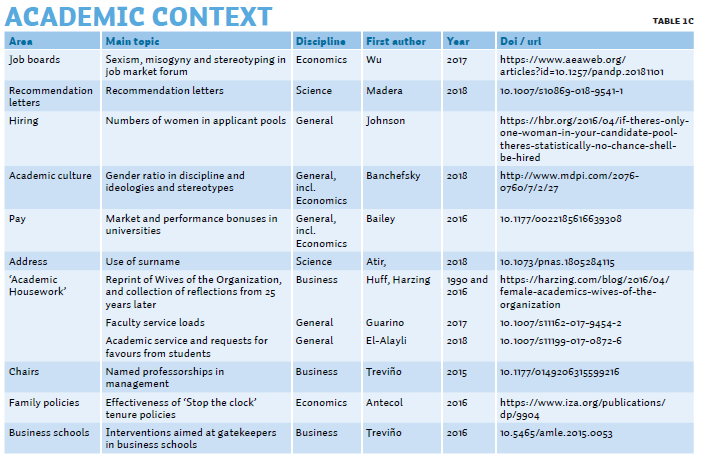
References
Arensbergen, P. van, I. Bleijenbergh and M. Lansu (2017) Gender training implementation report. Available at www.egera.eu.
Abraham, M. (2017) Pay formalization revisited: considering the effects of manager gender and discretion on closing the gender wage gap. Academy of Management Journal, 60(1), 29–54.
Besselaar, P. van den, and U. Sandström (2016) Gender differences in research performance and its impact on careers: a longitudinal case study. Scientometrics, 106(1), 143–162.
Besselaar, P. van den, and U. Sandström (2017) Vicious circles of gender bias, lower positions, and lower performance: gender differences in scholarly productivity and impact. PLoS ONE, 12(8), e0183301.
Biernat, M. and A.K. Sesko (2018) Gender stereotypes and stereotyping: a cognitive perspective on gender bias. In: N. Dess, J. Marecek and L. Bell (eds.), Gender, sex, and sexualities. New York: Oxford University Press, 172–191.
Biernat, M., M.J. Tocci and J.C. Williams (2011) The Language of performance evaluations: gender-based shifts in content and consistency of judgment. Social Psychological and Personality Science, 3(2), 186–192.
Bleijenbergh, I.L., M.L. van Engen and C.J. Vinkenburg (2013) Othering women: fluid images of the ideal academic. Equality, Diversity and Inclusion: An International Journal, 32(1), 22–35.
Bleijenbergh, I.L. and M.L. van Engen (2015) Participatory modeling to support gender equality: the importance of including stakeholders. Equality, Diversity and Inclusion: An International Journal, 34(5), 422–438.
Bosquet, C., P.-P. Combes and C. García-Peñalosa (2018) Gender and promotions: evidence from academic economists in France. Scandinavian Journal of Economics, forthcoming.
Brink, M. van den (2015) The politics of knowledge: the responses to feminist research from academic leaders. Equality, Diversity and Inclusion: An International Journal, 34(6), 483–495.
Castilla, E.J. (2015) Accounting for the gap: a firm study manipulating organizational accountability and transparency in pay decisions. Organization Science, 26(2), 311–333.
Castilla, E.J. and S. Benard (2010) The paradox of meritocracy in organizations. Administrative Science Quarterly, 55(4), 543–676.
Devine, P.G., P.S. Forscher, W.T.L. Cox et al. (2017) A gender bias habit-breaking intervention led to increased hiring of female faculty in STEMM departments. Journal of Experimental Social Psychology, 73, 211–215.
Dijk, H. van, B. Meyer and M. van Engen (2018) If it doesn’t help, it doesn’t hurt? Information elaboration harms the performance of gender diverse teams when attributions of competence are inaccurate. PLoS ONE, 13(7), e0201180.
Fang, F.C. and A. Casadevall (2016) Research funding: the case for a modified lottery. mBio, 7(2), e00422–16.
Goldin, C.A. (2014) A grand gender convergence: its last chapter. American Economic Review, 104(4), 1091–1119.
Handley, I.M., E.R. Brown, C.A. Moss-Racusin and J.L. Smith (2015) Quality of evidence revealing subtle gender biases in science is in the eye of the beholder. Proceedings of the National Academy of Sciences, 112(43), 13201–13206.
Harzing, A.W. (2007) Publish or perish. Available at harzing.com.
Harzing, A.W. (2014) CYGNA, supporting women in academia network. Available at harzing.com.
Harzing, A.W. and W. Mijnhardt (2015) Proof over promise: towards a more inclusive ranking of Dutch academics in Economics & Business. Scientometrics, 102(1), 727–749.
Heilman, M.E. (2012) Gender stereotypes and workplace bias. Research in Organizational Behavior, 32, 113–135.
Hsieh, C.-T., E. Hurst, C.I. Jones and P.J. Klenow (2013) The allocation of talent and U.S. economic growth. National Bureau of Economic Research. Available at klenow.com.
Joshi, A., B. Neely, C. Emrich et al. (2015) Gender research in AMJ: an overview of five decades of empirical research and calls to action: thematic issue on gender in management research. Academy of Management Journal, 58(5), 1459–1475.
King, E.B. (2006) The effect of bias on the advancement of working mothers: disentangling legitimate concerns from inaccurate stereotypes as predictors of career success. PhD thesis, Rice University. Available at scholarship.rice.edu.
King, E.B., M.R. Hebl, J.M. George and S.F. Matusik (2010) Understanding tokenism: Antecedents and consequences of a psychological climate of gender inequity. Journal of Management, 36(2), 482–510.
Koenig, A.M., A.H. Eagly, A.A. Mitchell and T. Ristikari (2011) Are leader stereotypes masculine? A meta-analysis of three research paradigms. Psychological Bulletin, 137(4), 616–642.
Lee, R. van der, and N. Ellemers (2015) Gender contributes to personal research funding success in The Netherlands. Proceedings of the National Academy of Sciences, 112(40), 12349–12353.
Leslie, S.-J., A. Cimpian, M. Meyer and E. Freeland (2015) Expectations of brilliance underlie gender distributions across academic disciplines. Science, 347(6219), 262–265.
Martell, R.F., C.G. Emrich and J. Robison-Cox (2012) From bias to exclusion: a multilevel emergent theory of gender segregation in organizations. Research in Organizational Behavior, 32, 137–162.
McKinsey (2018) Capturing the potential: advancing gender equality in the Dutch labor market. McKinsey Global Institute. Available at www.mckinsey.com.
Milkman, K.L., M. Akinola and D. Chugh (2015) What happens before? A field experiment exploring how pay and representation differentially shape bias on the pathway into organizations. Journal of Applied Psychology, 100(6), 1678–1712.
Miller, D.I., A.H. Eagly and M.C. Linn (2015) Women’s representation in science predicts national gender-science stereotypes: evidence from 66 nations. Journal of Educational Psychology, 107(3), 631–644.
Miller, D.I., K.M. Nolla, A.H. Eagly and D.H. Uttal (2018) The development of children’s gender-science stereotypes: a meta-analysis of 5 decades of U.S. Draw‐A‐Scientist Studies. Child Development, in press. Available at onlinelibrary.wiley.com.
Moss-Racusin, C.A., J.F. Dovidio, V.L. Brescoll et al. (2012) Science faculty’s subtle gender biases favor male students. Proceedings of the National Academy of Sciences of the United States of America, 109(41), 16474–16479.
Moss-Racusin, C.A., A.K. Molenda and C.R. Cramer (2015) Can evidence impact attitudes? Public reactions to evidence of gender bias in STEM fields. Psychology of Women Quarterly, 39(2), 194–209.
Nielsen, M.W. (2016) Limits to meritocracy? Gender in academic recruitment and promotion processes. Science and Public Policy, 43(3), 386–399.
Ridgeway, C.L. (2009) Framed before we know it. Gender & Society, 23(2), 145–160.
Rudman, L.A. and J.E. Phelan (2008) Backlash effects for disconfirming gender stereotypes in organizations. Research in Organizational Behavior, 28, 61–79.
Sarsons, H. (2017) Recognition for group work: gender differences in academia. American Economic Review: Papers and Proceedings, 107(5), 141–145.
Schein, V. E. (1973) The relationship between sex-role stereotypes and requisite management characteristics. Journal of Applied Psychology, 57(2), 95–100.
Teunissen, S. and C. Hogendoorn (2018) Too few women in economic debate.ESB, 103(4767S), 6-9.
Vinkenburg, C.J. (2017) Engaging gatekeepers, optimizing decision making, and mitigating bias: design specifications for systemic diversity interventions. The Journal of Applied Behavioral Science, 53(2), 212–234.
Vinkenburg, C.J., M.L. van Engen and P. Peters (2015) Promoting new norms and true flexibility: sustainability in combining career and care. In: A. de Vos en B.I.J.M. van der Heijden (eds.), Handbook of Research on Sustainable Careers. London: Edward Elgar.
Witteman, H.O., M. Hendricks, S. Straus and C. Tannenbaum (2017) Female grant applicants are equally successful when peer reviewers assess the science, but not when they assess the scientist. Available at www.biorxiv.org.