To prevent data-driven markets from tipping towards monopoly, policy makers should consider the introduction of data-sharing requirements in specific sectors. Inspiration on how to reconcile the interests of competition, innovation and data protection can be drawn from legislative initiatives.
In short
– Data sharing has positive welfare effects in markets characterized by strong data-driven indirect network effects.
– Ongoing policy initiatives illustrate a trend towards opening up privately held datasets, beyond competition enforcement.
– Empirical research is necessary to determine which sectors would benefit from mandatory data sharing.
In policy circles, the role of data as key enabler of growth is gaining attention. The European Commission aims to stimulate innovation by incentivizing sharing and reusing data within its European Data Economy initiative (European Commission, 2017). In ESB’s New Year’s article, the Dutch Ministry of Economic Affairs similarly recognized that the innovative use of data as well as its contribution to society will increase by making data more widely available.
In particular, the Ministry adopted the data-sharing proposal put forward by Prüfer and Schottmüller in their paper Competing with Big Data: “By increasing access to such anonymized clickstream data, other parties in different markets can use them for further innovation. At the same time, a strong concentration of large internet companies on these markets can be avoided (Prüfer and Schottmüller, 2017).” “One can think of the markets for digital maps, retail and, in the future, autonomous cars.” (Camps, 2018). Following the increasing support for data sharing among policy makers, this essay revisits the central arguments of Prüfer and Schottmüller (2017) (henceforth: PS) to explain why and when data sharing is essential. In addition, we discuss policy responses and implementation options for mandated data sharing.
Competing with big data: looking back
PS introduced data-driven indirect network effects as a novel economic mechanism, defined data-driven markets, and thereby clarified which type of data – namely user information – are “the oil of the data economy” (The Economist, 2017).
User information is data about the preferences or characteristics of a service’s users, for instance, the geographic location of a mobile app user or the revealed taste of a search-engine user looking for Sicilian restaurants in Amsterdam. These data are private information of the provider who collected it and can be used as innovation input in adapting the product better to users’ preferences, thereby increasing perceived quality in the future.
Thus, higher initial demand of a provider reduces marginal cost of innovation: it is cheaper to produce one additional unit of product or service quality, as perceived by users. This economic mechanism is called data-driven indirect network effects, and markets that are subject to these effects are called data-driven markets. Crucially, collecting user information is a byproduct of the search and transactions procedure that is virtually free for service providers. This creates a barrier to enter the market for would-be competitors.
By contrast, markets that require positive marginal cost to collect data about users or where the subject of the data is not users’ preferences or characteristics, are not data-driven, in the sense of the model. In these markets data may also be important, but its impact follows better known economic mechanisms, and market tipping is usually less strong.
The central result of the paper showed that data-driven markets nearly always tip, moving towards monopoly. Crucially, innovation incentives in a tipped market are low. Challengers compete against an incumbent with low marginal cost of innovation due to high user information stocks, and the dominant firm knows of the fear of potential challengers due to its data advantage. Hence, after market tipping not only competition in the market but also competition for the market is heavily reduced.
This theoretical result is supported empirically (He et al., 2017). Summarizing their empirical study on search engine quality, Preston McAfee, the Chief Economist of Microsoft, recently referred to the key mechanism: “Even at web scale, more data makes search better.” (McAfee, 2015). Junqué de Fortuny et al. (2017) confirm that data has diminishing returns but a strictly positive impact on the precision of prediction analytics. Hence, the additional value of more data on user preferences and characteristics gets smaller if one already has a lot of data – but the value is always positive and it matters for quality and, hence, market outcomes.
PS also introduced the idea of connected markets, to rationalize business strategies of the most successful global firms. Providers can connect markets if the user information they have gained is also valuable in another market. For instance, some search engine queries relate to geographical information. Such data is also valuable when providing a customized map service. PS showed that, if market entry costs in a ‘traditional’ market are not too high, a firm that finds a ‘data-driven’ business model can dominate any market in the long run. Relevant user information on its home market is a great facilitator in this process, which can occur repeatedly, generating a domino effect across markets.
Indirect network effects
Critics might say that we’ve heard this before and that data-driven indirect network effects are the same as learning-curve effects. Ten years ago pessimists wrongly predicted the eternal dominance of Microsoft. The difference is that in the learning-curve literature, if two competitors have about equal experience (= previous output), they both have about equal production costs and, hence, will share a market equally, forever. Moreover, learning curves are typically temporary advantages. As soon as a firm hits the technological frontier, its learning curve advantage will steadily decline and entrants or smaller firms can catch up.
In a data-driven market, however, firms’ choice variable is investment in quality enhancement, instead of prices, and therefore differences between firms are permanent once both firms find it optimal not to invest from a certain point of time onwards – which occurs after market tipping. An entrant or smaller player cannot profitably make the quality investment necessary to equal the incumbent.
The key novelty from a competition policy perspective is that in a market where a learning curve may apply, say in aircraft manufacturing, smaller firm B can poach dominant firm A’s key engineers – thereby acquiring relevant knowledge – and fiercely compete with firm A. In data-driven markets, even if firm B poaches firm A’s key software/algorithm developers, firm A still retains its user information, preserving A’s lower cost of innovation.
This robustness of the dominant position in a data-driven market is also different from Microsoft’s dominance in the desktop operating systems market, which is based on direct network effects. The latter are a powerful force of dominance but can be overcome by a better product, as Myspace had to learn from Facebook. If a dominant firm in a data-driven market would compete with a radically innovative, ‘better’ product, it could mimic the market entrant at much lower cost of innovation because of its extensive and exclusive knowledge of user preferences and characteristics.
Two types of policy responses are available to address the monopolization tendency in data-driven markets, namely: competition enforcement and regulation. Although regulation can complement competition law, new regulatory interventions should only be considered when competition enforcement is not sufficient to remedy the identified concerns.
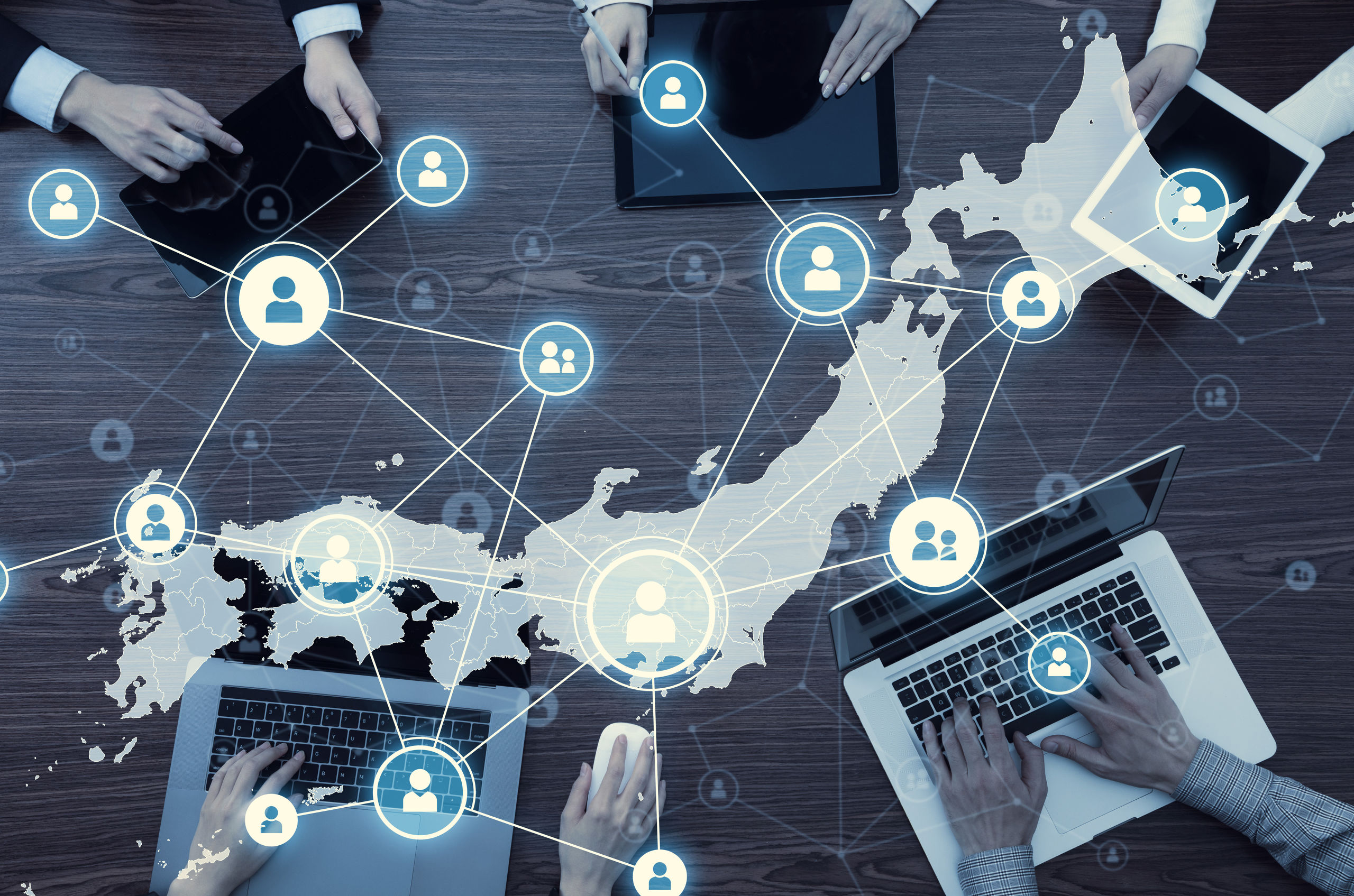
Competition enforcement
Data is a non-rival good: if one entity has used a piece of data, this does not preclude others from using identical information. Furthermore, value from data does often not derive from the collected information itself, but from the knowledge that can be extracted from it. This implies that different entities may generate the same knowledge by gathering distinct datasets.
However, claims about the wide availability of data that are often raised by opponents of mandated data sharing need to be nuanced. For launching a particular market activity, a specific type of data is needed that may not be readily available on the market and may neither be replicable by a new entrant. For example, if the specific data needed to operate a search engine of good quality can only be obtained through serving customers, other data available from third parties will not form an adequate substitute for the search data of the incumbent (Graef, 2016).
Under competition law, the relevant question to be answered is whether abuse of dominance can be established, which would enable competition authorities to intervene in the market. When a dominant firm denies access to user information that a competitor needs in order to develop its own service, such a refusal to deal can in certain circumstances amount to abuse in breach of Article 102 of the Treaty on the Functioning of the European Union.
The key requirement for establishing competition liability for refusals to give access to user information is its indispensability as an input. According to the Bronner judgment of the European Court of Justice, indispensability requires the existence of technical, legal or even economic obstacles capable of making duplication impossible, or even unreasonably difficult. It would be necessary at the least to establish that it is not economically viable to create an alternative facility at a scale comparable to that of the dominant firm.
This raises the question whether data generated in markets characterized by strong indirect network effects, like search engines and social networks, is more likely to be regarded as indispensable, as it is virtually impossible for third parties to build up an equally large and varied dataset based on the economic features of the market. For instance, given that Facebook has such detailed information that it can divide its users in no less than 29,000(!) categories, entrants can hardly compete on the merits (ProPublica, 2016).
Regulation
While competition law can thus be invoked to gain access to the dataset of a dominant firm, its scope is limited. Access can only be imposed on a case-by-case basis, namely in the specific circumstances where a refusal to deal amounts to abuse. A regulatory intervention beyond competition law requires the weighing of different public interests, including competition, innovation and data protection.
Innovation
As regards competition and innovation, there is a trade-off between the short- and long-term impact of a regulatory intervention. Mandated data sharing will increase competition and innovation in the short run, but too restrictive regulation may harm competition and innovation in the long run. Some fear that dominant firms may lose incentives to invest in new facilities. Competitors, in turn, may no longer be stimulated to develop alternative facilities if they have guaranteed access to the dominant firm’s data.
Tackling this argument, PS studied the consequences of a regulatory requirement for dominant firms in data-driven markets to share their (anonymized) user information with one another, based on the earlier idea of Argenton and Prüfer (2012). The outcome shows that, even in a dynamic model where competitors know that their innovation investments today affect their market shares and hence their innovation costs tomorrow, such a policy intervention would have positive net effects on innovation and welfare if data-driven indirect network effects are sufficiently strong in that market. Both the dominant firm and its competitors would have stronger incentives to innovate under a regime of data sharing than under the status quo: if an entrant gets access to the dominant firm’s dataset on user information, it still has to develop the analytical tools to learn something valuable from these data – and to customize its product to users’ needs.
Competition
Although one may claim that many digital giants can be regarded as innovative companies, there is no evidence that more competition in data-driven markets reduces innovation. Established empirical research has shown that under an inverted-U curve increased competition only leads to more innovation up until a certain point after which innovation diminishes, as competition further intensifies (Aghion et al., 2005). However, because of their concentrated nature, data-driven markets would find themselves on the far left side of the inverted-U, where an increase in competition predicts a stimulus of innovation.
Data protection
Simultaneously, the fundamental right to data protection needs to be considered, as the sharing of user information is likely to relate to identified or identifiable natural persons. Anonymizing data before sharing may offer a solution. However, because of ever stronger technological possibilities to re-identify individuals, it seems hard to claim that datasets can be perfectly anonymous – but data protection concerns should not be used as an excuse not to intervene at all.
The right to data portability, recently introduced by the General Data Protection Regulation (GDPR), entitles data subjects to transfer personal data from one data controller to another in a structured, commonly used and machine-readable format. This new right may foster competition as it reduces lock-in by enabling users to switch easily between services. One can even imagine that market players will use it proactively by trying to convince data subjects to request data from their previous provider (and offer them discounts). This relates to the idea that data generation should be regarded as labor that should be paid for (Arrieta-Ibarra et al., 2018).
Yet, the GDPR does not require data controllers to delete personal data once the data subject makes such a data-portability request. Consequently, a dominant firm, even if transferring some user information to competitors following user requests, would still have access to more data than its competitors. The data subject may also invoke the right to erasure but, due to its limited scope of application, the alignment between these two independent rights is not perfect (Graef et al., 2017).
In many situations, data controllers will thus be able to continue relying on ported personal data to improve their services. However, there is a positive externality from switching: for every new user, other users of the new provider will get better predictions. As users typically do not take this benefit into account when deciding whether to move their data, we should expect too little switching. Therefore, the right to data portability – even if widely invoked by data subjects – is unlikely to remedy market tipping. Nevertheless, its inclusion as a regulatory tool encouraging sharing of personal data under data protection law does illustrate that the interests of innovation and data protection are not incompatible per se.
Mandated data sharing: looking ahead
To implement a data-sharing regime, inspiration can be drawn from ongoing efforts in specific sectors. The most instructive parallel can be drawn with the Payment Services Directive 2 (PSD2) in the financial industry, which entitles third parties, with the consent of the account holder, to access payment accounts in order to initiate payment transactions via an internet application or to consolidate account information from one or more accounts into one application. As such, PSD2 is a perfect example of regulation by the EU legislator to level the playing field in the financial sector. Now, banks have to accommodate fintech start-ups offering innovative services. For implementing access to an account, the European Banking Authority adopted several Guidelines and Regulatory Technical Standards to clarify the steps banks need to take, and several initiatives are defining common API standards.
In other industries, policy initiatives to create more openness are also visible. The European Commission reportedly received concerns about data access in the agricultural and automotive sectors, where stakeholders are worried that the required data remains under the control of manufacturers to the detriment of the development of, respectively, ‘smart farming’ and new uses based on in-vehicle data.
All these policy initiatives show the gradual shift towards opening up markets with the aim of stimulating innovation, whereas the idea of mandated data sharing is developed further by academics, including Mayer-Schönberger and Ramge (2018): “Building on this idea [of Prüfer and Schottmüller], we suggest what we term a progressive data-sharing mandate. It would kick in once a company’s market share reaches an initial threshold – say, 10 percent. It would then have to share a randomly chosen portion of its feedback data with every other player in the market that requests it. How much data it must make available would depend on the market share captured by the company. The closer a company is to domination, the more data it would have to share with its competitors.”
A complementary question as to how to organize data sharing among competitors, is which markets exactly should be subjected to data sharing. PS showed that in markets where data-driven indirect network effects are important, mandated data sharing has positive net welfare effects, whereas this is less clear if these effects only play a minor role. As an illustration of this, it is conceivable that indirect network effects are of major importance in markets for search engines and for social networks. By contrast, in retail electricity markets, where smart meters also allow energy suppliers to collect exclusive information about buyers’ consumption patterns at household level, other dimensions of innovation may be more important for market success.
Conclusion
Notably, these examples are based on intuition and not on solid empirical research. Consequently, the most important next step towards a policy restricting the negative effects of monopolization on data-driven markets is to conduct a series of industry studies establishing empirically which markets are more and which are less data-driven and, hence, where mandatory data sharing would spur welfare and avoid diminished innovation incentives.
References
Aghion, P., N. Bloom, R. Blundell et al. (2005) Competition and innovation: an inverted-U relationship. The Quarterly Journal of Economics, 120(2), 701–728.
Argenton, C. and J. Prüfer (2012) Search engine competition with network externalities. Journal of Competition Law and Economics, 8(1), 73–105.
Arrieta-Ibarra, I., L. Goff, D. Jiménez-Hernández et al. (2018) Should we treat data as labor? Moving beyond ‘Free’. AEA Papers and Proceedings, 108, 38–42.
Camps, M. (2018) New year’s article 2018: maintaining focus amid blurring boundaries. Article at esb.nu.
European Commission (2017) Communication from the Commission to the European Parliament, the Council, the European Economic and Social Committee and the Committee of the Regions, ‘Building a European Data Economy’, 10 January, COM(2017) 9 final.
Graef, I. (2016) EU competition law, data protection and online platforms: data as essential facility. Alphen a/d Rijn: Kluwer Law International.
Graef, I., M. Husovec and N. Purtova (2017) Data portability and data control: lessons for an emerging concept in EU law. TILEC Discussion Paper, 2017-041.
He, D., A. Kannan, R.P. McAfee et al. (2017) Scale effects in web search. International Conference on Web and Internet Economics – WINE 2017, 10660, 294–310.
Junqué de Fortuny, E., D. Martens and F. Provost (2013) Predictive modeling with big data: is bigger really better? Big Data, 1(4), 215–226.
Mayer-Schönberger, V. and T. Ramge (2018) Reinventing capitalism in the age of big data. London: John Murray.
McAfee, P., J. Rao, A. Kannan et al. (2015) Measuring scale economies in search. Slides at www.learconference2015.com.
ProPublica (2016) Facebook doesn’t tell users everything it really knows about them. Article at www.propublica.org.
Prüfer, J. and C. Schottmüller (2017) Competing with big data. CentER Discussion Paper, 2017-007.
The Economist (2017) The world’s most valuable resource is no longer oil, but data. The Economist, 6 May.
Auteurs
Categorieën